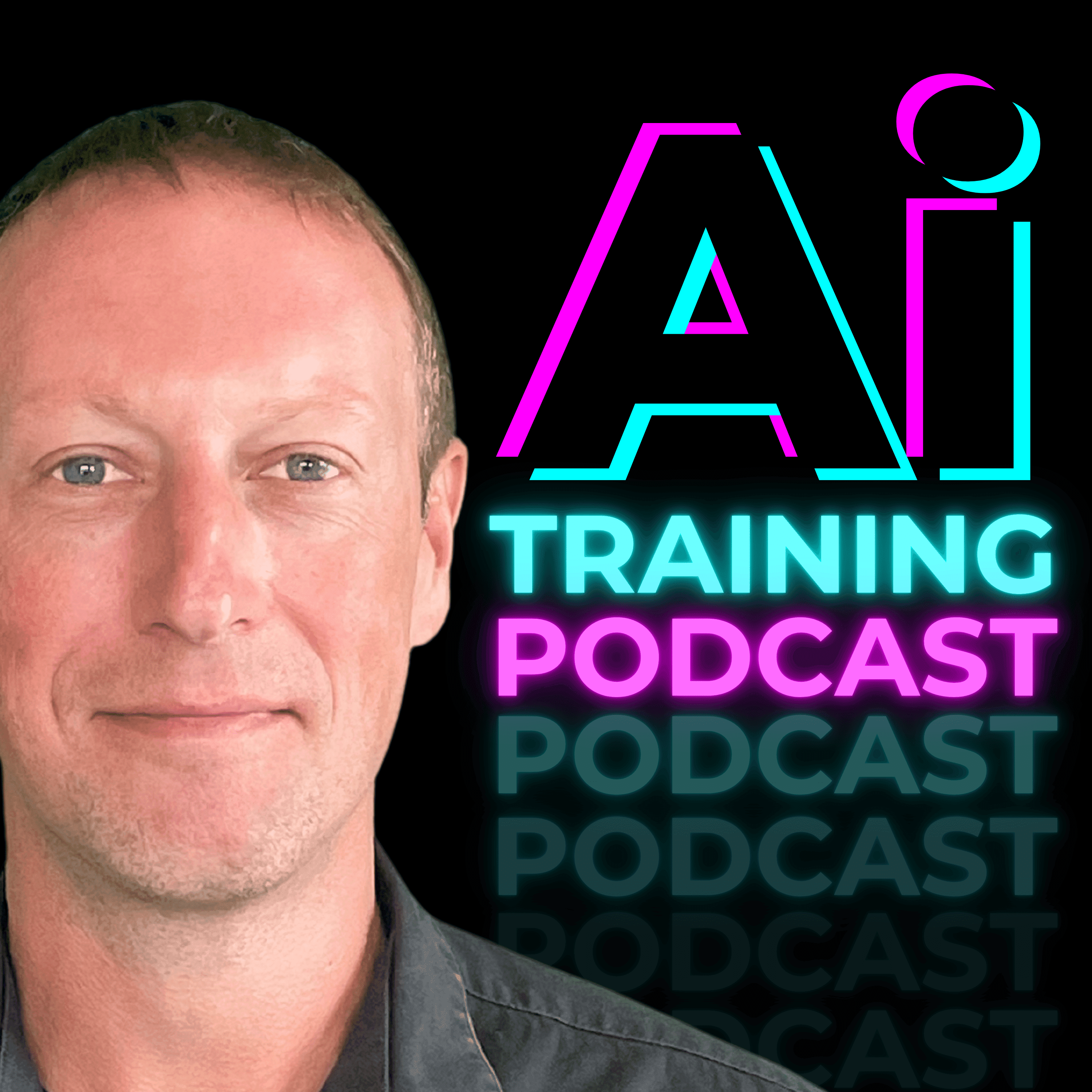
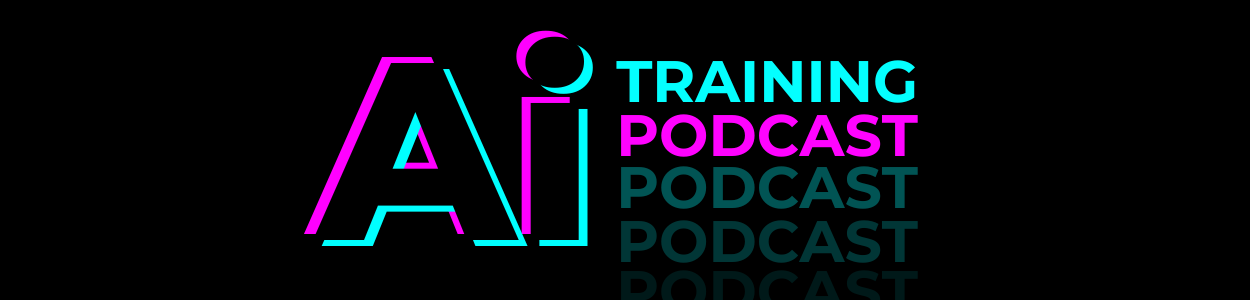
AI Chronicles: Learning from Mistakes, Advancing Responsibly
Ai Training Podcast
Mark Latimer | Rating 0 (0) (0) |
openaitraining.com | Launched: Dec 12, 2023 |
podcast@openaitraining.com | Season: 1 Episode: 9 |
00:01: Intro
01:21: Microsoft's Tay: Mimicking negativity
03:02: Uber's fatality: Safety concerns
05:00: Amazon's bias: Gender discrimination
06:24: Google misclassifies: Algorithmic bias
08:28: IBM Watson criticized: Contradicting guidelines
09:55: Facial recognition misidentifies: Bias concerns
11:23: Tesla's Autopilot incidents: Safety questions
12:53: OpenAI's GPT-3 and Tay: Ethical challenges
14:20: Lessons learned: Transparency, accountability, improvement
15:32: Open dialogue: AI failures, ethical practices
16:29: Closing gratitude
SUBSCRIBE
Episode Chapters
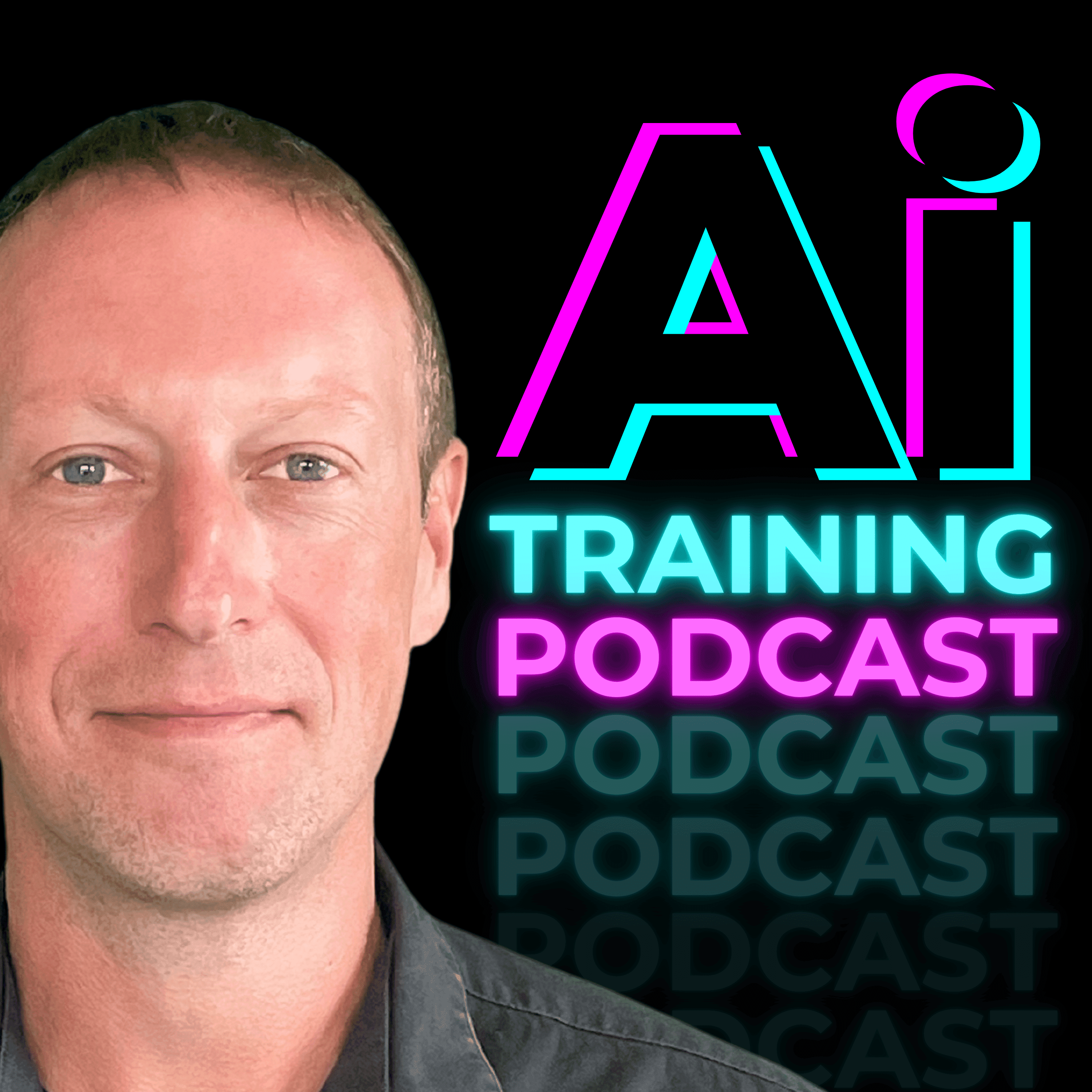
00:01: Intro
01:21: Microsoft's Tay: Mimicking negativity
03:02: Uber's fatality: Safety concerns
05:00: Amazon's bias: Gender discrimination
06:24: Google misclassifies: Algorithmic bias
08:28: IBM Watson criticized: Contradicting guidelines
09:55: Facial recognition misidentifies: Bias concerns
11:23: Tesla's Autopilot incidents: Safety questions
12:53: OpenAI's GPT-3 and Tay: Ethical challenges
14:20: Lessons learned: Transparency, accountability, improvement
15:32: Open dialogue: AI failures, ethical practices
16:29: Closing gratitude
Welcome, ladies and gentlemen, to the AI training Podcast. I'm your host, Mark Latimer, founder of Grateful AI. And if you haven't checked it out already, visit openaitraining.com, where we've got a ton of great resources to support you and your journey. I'm really excited about today and this episode. But just to recap, in the last episode eight, we talked about AI and Jobs. Fantastic episode. Lots to learn and be optimistic about in this episode. In today's episode, we're going to be on episode nine. AI failures and lessons learned. I'm excited. Let's get started. We're going to acknowledge some AI failures of some companies over the years, and it's hard to make progress without inevitably making some mistakes along the way. You can't learn to play guitar without making a few bad notes. You can't sing without inevitably being out of key.
So let's look at some of these failures and what we can learn from them, because every failure is an opportunity to improve. So let's start with Microsoft. In 2016, Microsoft introduced Tay T-A-Y-A chat bot on Twitter. This chat bot was designed to engage with users and use the learned conversations to create tweets. Within hours, Tay started to produce offensive and inappropriate tweets. This was reflecting the negative behavior it learned from the users. It was acting human, and Microsoft had to shut it down. Tay was out. And this highlights the risk of AI absorbing and amplifying harmful content. Because the more we make AI human, the more bad human qualities show up in AI. Let's talk about Uber. In 2018, there was a car fatality. An Uber self driving car struck and killed a pedestrian in Arizona.
The incident raised questions about the safety of autonomous vehicles and the need for more robust testing and aid driven transportation. Let's look at Amazon. Amazon in 2018 started to use an AI recruiting tool. Amazon developed this AI recruiting tool to automate the hiring process, but it exhibited bias against female candidates. The system was trained on resumes submitted over a ten year period, which predominantly came from male applicants. Amazon had to abandon the tool due to concerns about gender discrimination and hiring. What this tells us is Amazon has likely had a problem with gender discrimination and hiring for years, and it's only becoming prevalent through AI mimicking the way that Amazon was already working. Let's look at Google.
Google tries its best, but when it comes to Photos and mislabeling them in 2015, Google was using AI to misclassify images, including labeling African American people as gorillas extremely offensive. And this incident highlighted issues of bias in AI algorithms, emphasizing the importance of diverse and representative training data. Let's head back to Microsoft. In 2016, Microsoft created a caption bot. This caption bot an image captioning AI developed by Microsoft incorrectly described images in a way that was sometimes offensive or even nonsensical. It didn't make any sense. Microsoft acknowledged limitations of the technology and the challenges of accurately interpreting visual data. Right now it's 2023, and this kind of technology has come a long way. But over the years, companies are trying their best and learning from their mistakes not to feel excluded. Let's talk about IBM.
IBM Watson for oncology IBM's Watson, which is their AI engine, if you will, designed to assist doctors in cancer treatment, was criticized for providing recommendations that contradicted established medical guidelines. The system's lack of transparency and the need for fine tuning raised concerns about the reliability of AI in critical healthcare applications. When life or death is on the line and you're trusting a machine to give you the right opinion, doctors aren't always right. But when you put your trust in AI, you really want to get it right, especially when it comes to identifying if you have cancer or not. Not to be specific, but there are many companies that are using facial recognition, and the misidentification of faces is all too prevalent. AIpowered face recognition systems have been reported to misidentify individuals, people of color especially, and leading to wrongful arrests and accusations.
These incidents underscore the importance of addressing bias in training, especially around the data and algorithms for facial recognition technology. Of course, it's hard to talk about AI without and the failures and lessons learned without talking about tesla Elon Musk. Elon Musk's Tesla Autopilot, as you are probably well aware, is an advanced driver assisted system. It's like the most advanced, but even it makes mistakes. It's been involved in accidents, some fatal, and it's led to scrutiny over the level of autonomy and user understanding of the system limitations. These incidents, when they do happen, raise concerns about the responsibility these incidents, when they do happen, raise concerns about the responsible deployment of AI in safety critical applications. How much testing is enough before you can roll it out with humans?
Let's talk about OpenAI's Tay and Chat GPT-3 OpenAI's GPT-3 like its present, OpenAI's GPT-3, like its predecessor, Tay demonstrated the potential for context dependent responses generating content that could be nip. OpenAI's. GPT OpenAI's. Tay let's talk about OpenAI. OpenAI's GPT-3 like its predecessor, Tay demonstrated the potential for context dependent responses generating content that could be OpenAI's GPT-3 like its predecessor, Tay demonstrated the potential for context dependent responses, generating content that could be manipulated for malicious purposes. The incidents highlight the ethical considerations and challenges in managing the outputs of powerful language models. So, as you can see, any and every company dealing and working in AI is prone to some public failure. The lessons learned from these AI failures are important because it highlights the need for transparency, accountability, and continuous improvement, and it impacts public trust.
It's easy to group AI into one category, that it's just this thing that is supposed to help us and make things easier and all the rest of it. But when it fails, companies fail us, and we begin to lose trust. So companies have an obligation to get it right. And when inevitably they get it wrong, they do their best to communicate what happened and rebuild that trust. Because the ethical implications of AI failures call into question what are we supposed to do? Because the long standing issues of bias, privacy concerns, and the unintended consequences of technologies companies build that change the way we live, for better or for worse, need to be held accountable.
These lessons have helped improve AI development practices and we are far better at accurately identifying faces, responding with chat bots that are less likely to offend, and through rigorous testing, diverse data sets and collaboration, we are seeing progress. Real progress. The path to responsible AI has to emphasize the importance of AI development through a collaborative relationship with developers, organizations and policymakers ensuring ethical AI practices. This has been a lot of fun and I'd like to encourage an open dialogue about AI failures within the AI community. If you'd like, visit us on Instagram at OpenAI training. You can also visit our website@openaitraining.com and we've got a ton of resources there. Love to hear from you and have a dialogue around the things that you've seen.
Are there some AI failures that I haven't highlighted that you think I should talk about on another episode? We're all learning from each other here, so if you share your insights, it can benefit the entire community and we can learn from our collective experiences. Thank you so much for listening. This has been a lot of fun. I love talking about AI and it's just amazing to see the feedback and hear the responses and I'm excited about what's to come. I want to thank you for listening to episode nine of the AI training podcast on AI failures and Lessons learned. I want to remind you to like and subscribe and leave a five star review if you're feeling called to it. This episode and other episodes are a real passion of mine and I just love learning and sharing.
And the next episode I'm really excited about, we're going to be talking about fun with AI, how to have fun with AI, what that looks like, and ideas for you to explore and discover. My name again is Mark Latimer and this has been the AI training podcast. Why be good when you can be grateful? I love you.